4 Case Studies Highlight AI’s Role in Improving Pharmacy Benefit Performance
4 Case Studies Highlight AI’s Role in Improving Pharmacy Benefit Performance
October 18, 2023
See original article published by Kate Baggaley on Pharmacy Practice News
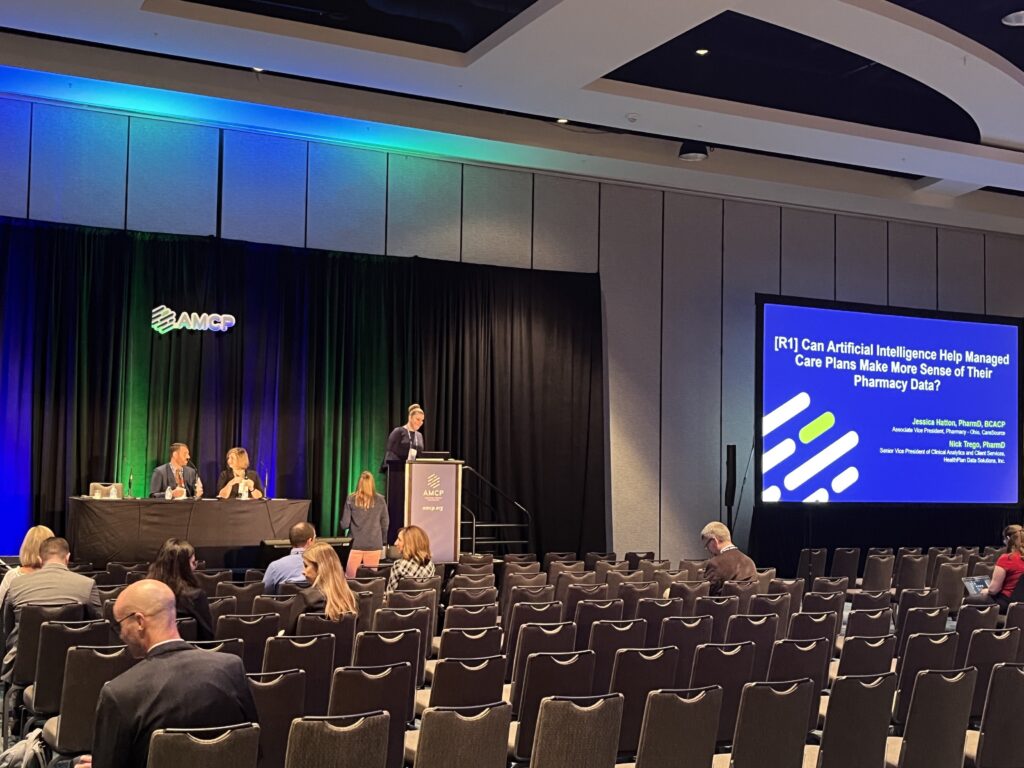
Artificial intelligence and machine learning can flag claims errors, prevent fraud, waste and abuse and streamline benefit contract reviews, speakers noted during a session at the AMCP Nexus 2023 meeting.
“We have 100% of our claims run through a technology platform”—a process that has yielded many of the benefits cited above, said Jessica Hatton, PharmD, BCACP, the associate vice president of pharmacy at CareSource, a nonprofit managed care organization, serving more than 2 million members. The contract review capabilities of AI are particularly useful when working with pharmacy benefit managers (PBMs), Dr. Hatton noted. “Contracts can be really long—hundreds to thousands of pages,” she said. These documents are also very complex, and “today most of the review is manual.”
But AI can expedite the review process. “[Computer] models can be trained to identify specific elements of a contract if you’re looking for specialty, generic and brand pricing,” said Nick Trego, PharmD, the senior vice president of clinical analytics and client services at the software company HealthPlan Data Solutions, which is based in Columbus, Ohio. “And the digital contract repository provides a single hub for all contracts and amendments so you don’t have to go looking through a whole bunch of folders on your computer or on your company-shared website to find these things.” Another area where AI can shine is pharmacy claims monitoring. “Right now, it’s challenging because depending on the population, you can have … up to millions of claims per month, and claims are very complex,” Dr. Hatton said. “There are many variables that make up whether a claim leads to a ‘paid’ or ‘rejected’ status.”
AI can catch errors as they occur. “High-throughput technology platforms can rapidly re-adjudicate 100% of the pharmacy claims to ensure their accuracy within hours of being put into the system,” Dr. Trego said. “We can identify and resolve the errors as they occur, versus at the end of the year or the end of the quarter.” As noted, AI can also improve detection of fraud, waste and abuse. An algorithm can be trained to rule out certain “false-positive” provider or prescription characteristics—for example, a pulmonologist frequently prescribing the same drug for cystic fibrosis—“because there’s not a ton of drugs out there for that disease state,” Dr. Trego said. The algorithm can then home in on more suspicious transactions, such as a provider repeatedly prescribing the same dose of the same type of opioid.
Additional opportunities for AI within managed care include:
- member engagement and customer service (e.g., programming voice assistants and chatbots to answer common questions);
- enhanced predictive modeling for targeted outreach; and
- formulary management that accounts for a plan’s unique population makeup.
The population-level analyses “allow us to combine our pharmacy data with medical history and social determinants of health … to engage with our most at-risk patients, and we hope improve their quality of care and reduce financial impact of adverse events,” Dr. Hatton noted.
Dr. Hatton presented four case studies from CareSource, headquartered in Dayton, Ohio, to demonstrate the utility of machine learning, which is a subset of AI involving computer systems that can learn and adapt without following explicit instructions.
1. Insulin copay caps.
Several states have attempted to lower patient out-of-pocket costs by implementing laws regarding insulin copay limits. Copay limits and time requirements vary from state to state. “The machine learning system was implemented to provide oversight so we could ensure compliance to the state regulations and look for any errors in insulin claim adjudication,” Dr. Hatton said. The system quickly identified an incorrect use of copay limits that were being defined by calendar month rather than rolling 30 days. The affected claims were flagged in near real time.
“We were able to alert our PBM of the issue and then correct those claims and reimburse members who had been overcharged,” Dr. Hatton said.
2. National Average Drug Acquisition Cost (NADAC) pricing methodology.
When CareSource launched a new initiative to implement NADAC in the reimbursement process for independent pharmacies, Dr. Hatton said, millions of claims within a custom pharmacy network were affected. “We definitely needed a way to monitor those claims and ensure the success of this initiative,” she said.
CareSource worked with HealthPlan Data Solutions on a machine learning model to identify discrepancies in reimbursement. “The system did flag some claims with incorrect adjudication logic that ended up being an incorrect setup for 340B reimbursement,” Dr. Hatton said. Identifying the discrepancy “avoided about $1 million in cost that we would’ve either had to absorb or … recoup from the pharmacies, and that really would’ve made the initiative very unsuccessful.”
3. Benefit design.
CareSource implemented a cost exceeds maximum (CEM) limit for over-the-counter (OTC) products “that had pretty broad coverage,” Dr. Hatton said. “We wanted to keep it pretty wide open, but we wanted to ensure that the most cost-effective options were being selected at dispensing.” The organization used a machine learning model to monitor the new CEM based on National Drug Codes (NDCs). “After it went live, … we were seeing tons of claims go through above the cost maximum,” she continued. By correcting this error, Dr. Hatton said, CareSource secured plan adherence, realized $25,000 in plan savings and ensured a continuous alert system for high-cost NDCs was in place to prevent future errors.
4. COVID-19 testing mandates.
After the Centers for Medicare & Medicaid Services implemented a maximum of eight OTC COVID-19 tests per calendar month at no out-of-pocket cost to the patient, CareSource used a machine learning model to identify discrepancies in reimbursement. The algorithm flagged a pharmacy that was filling the maximum eight tests for every patient, regardless of whether the patients requested or needed them. CareSource contacted the pharmacy and promptly resolved the issue.
The speakers reported no relevant financial disclosures.
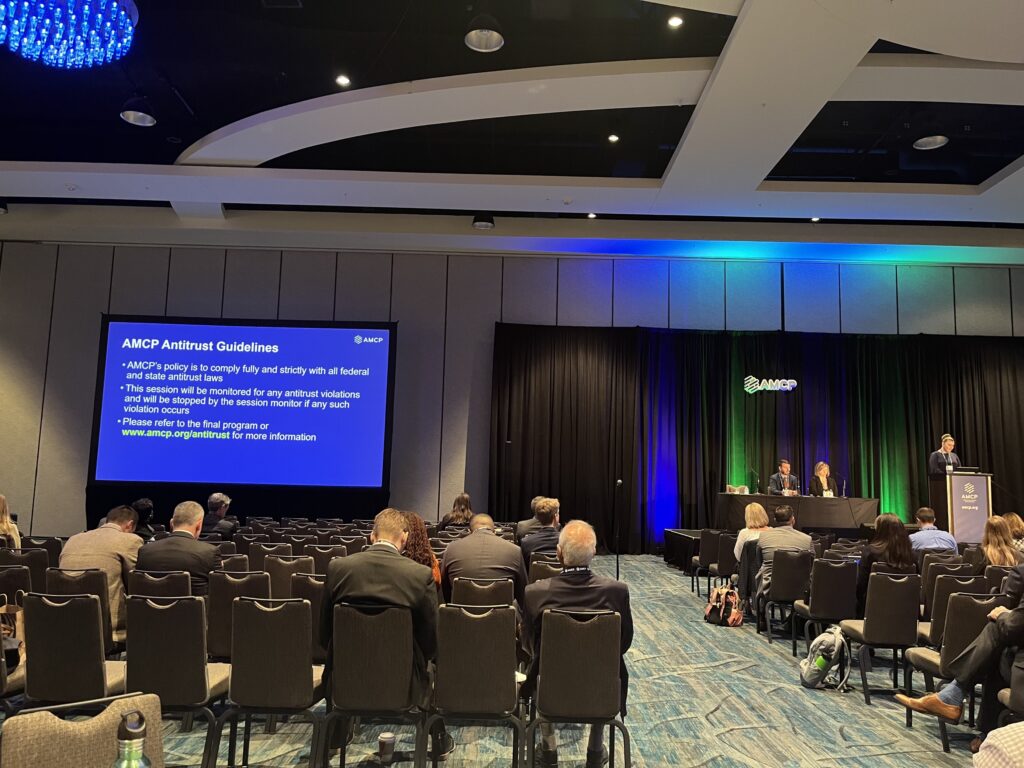
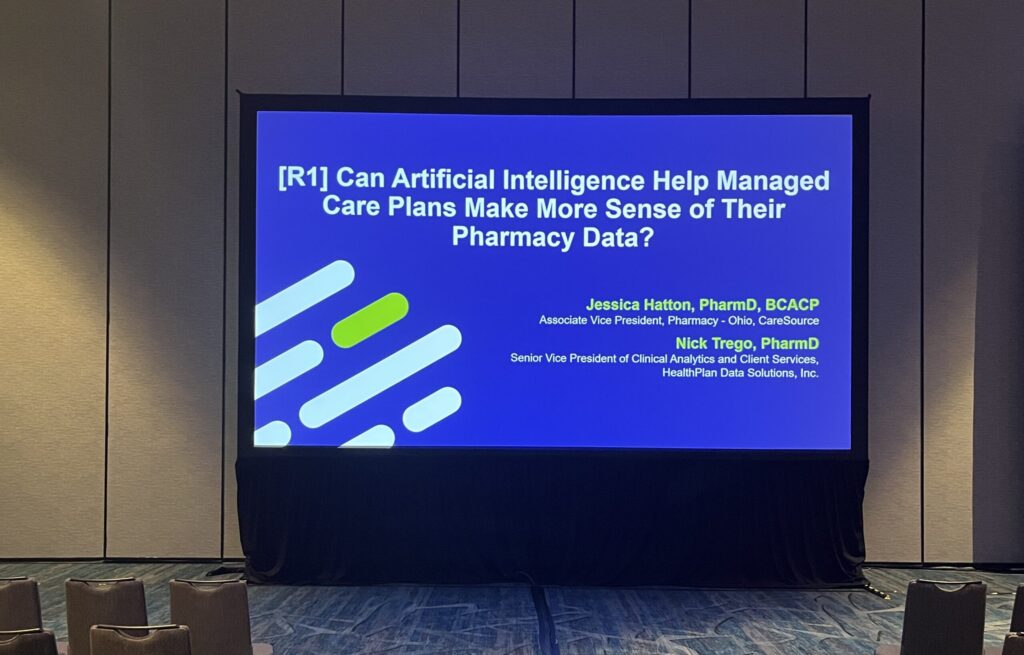